Latest Research Topics in Machine Learning for Scholars in 2024
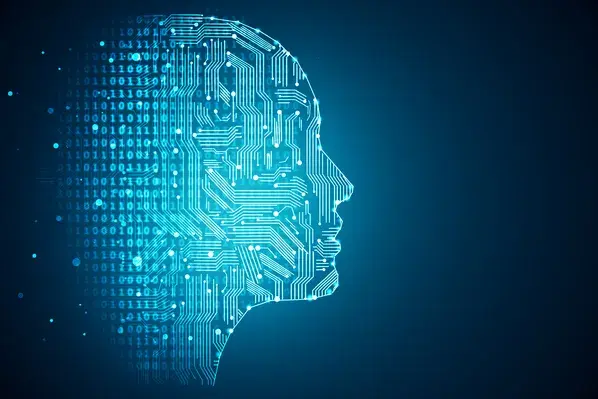
Machine Learning (ML) is advancing rapidly in 2024, transforming how we use computers and solve problems. This year, we are witnessing significant progress in how machines learn and think.
Many areas within ML are proliferating. Today, robots are becoming increasingly intelligent and are better able to understand language. Similarly, computers are now more adept at comprehending information. These advancements are driving positive change across various fields, including healthcare, finance, environmental science, and beyond.
Researchers are working hard to develop ML systems that need less data and consume less energy. They are also trying to make artificial intelligence (AI) that people can trust and understand.
Difference between AI and ML
Whereas AI focuses on simulating human-like intelligence and solving complex problems, ML (a subset of AI) uses data-driven algorithms to identify patterns and make predictions. AI encompasses various techniques, including ML, but can also operate without learning from data through rule-based systems or expert systems.
Key Trends in AI and ML Research for 2024:
- Making AI explain its decisions
- Teaching machines to learn like humans
- Using AI to fight climate change
- Keeping AI safe from attacks
For anyone interested in technology, it is a good time. Machine learning is opening new doors and solving old problems by adopting new approaches.
In this blog post, we will explore the key areas of ML research in 2024, highlighting the latest developments, significant trends, and potential future directions.
Foundational Research Topics
Presently, researchers are pushing into thrilling new areas and taking machine learning to the next level. They are solving complex problems and unlocking new possibilities. Here are some of the key areas researchers are focusing on this year.
Supervised Learning
Supervised learning remains a cornerstone of machine learning research. It involves training models on labeled data to make predictions or classifications. Recent advances include:
- Enhanced deep learning architectures for complex tasks
- Data-efficient algorithms that perform well with limited samples
- Improved model robustness and generalization techniques
These ML developments are impacting various sectors. For example, in healthcare, supervised learning models are enhancing disease diagnosis through medical imaging. Meanwhile, financial institutions are also utilizing these advanced predictive models for fraud detection and risk assessment.
Unsupervised Learning
Unsupervised learning focuses on identifying patterns in unlabeled data. Notable advancements in this area of machine learning research include:
- Advanced clustering algorithms that improve data grouping and classification.
- Innovative dimensionality reduction techniques that preserve essential features while simplifying data.
- Methods for uncovering hidden structures within large-scale datasets.
These advancements are proving valuable in multiple applications. Businesses are benefitting from improved customer segmentation for targeted marketing. In genomics, researchers are using enhanced clustering to identify gene expression patterns.
Reinforcement Learning
Reinforcement learning, which trains agents through interaction with environments, is seeing significant growth. Key developments include:
- Faster learning algorithms for complex robotic tasks
- Advanced game AI capable of human-level performance in strategic games
- Improved decision-making models for autonomous vehicles
These innovations are extending reinforcement learning's practical applications. Manufacturing is benefiting from more adaptable robotic systems.
Developers are creating more complex and realistic AI rivals in the gaming industry. Additionally, advanced machine learning algorithms are transforming the automotive industry as well by bringing in futuristic self-driving technology and seamless navigation.
As these foundational machine learning areas progress, they enable advancements in more specialized domains. They provide the building blocks for tackling increasingly complex problems and drive innovation across the AI landscape.
Advanced Research Areas
With advancing machine learning techniques and tools, researchers can now address complex challenges in less time. In 2024, three areas of advanced machine learning research stand out for their innovative approaches:
- Generative Adversarial Networks (GANs)
- Meta-Learning
- Featured Learning
GANs
GANs comprise two neural networks competing against each other, resulting in highly realistic artificial content. Recent innovations include:
- Improved stability in GAN training, leading to more consistent results
- Enhanced resolution and detail in generated images and videos
- Expansion into new domains such as 3D object generation and audio synthesis
GANs are finding diverse applications across industries. For instance, healthcare researchers are utilizing this to generate artificial medical images for training diagnostic systems.
On the other hand, in the entertainment industry, GANs are creating lifelike digital characters for movies and games, enhancing the overall experience. In the fashion industry, designers are now taking advantage of this technology to create new and unique textile designs.
Meta-Learning
Also known as "learning to learn," meta-learning focuses on building models that can adapt to new tasks quickly with minimal data. Key advancements include:
- Algorithms that can learn from just a few examples (few-shot learning)
- Models that rapidly adjust to new environments or problem domains
- Techniques for transferring knowledge across different but related tasks
This approach is particularly promising for applications where data is limited or quick adaptation is crucial. For instance, in robotics, meta-learning enables machines to learn new tasks swiftly. Besides, Natural Language Processing (NLP) enhances the ability of language models to understand and generate text in new contexts or languages.
Meta-learning also addresses the challenge of AI systems traditionally requiring large amounts of data and computational resources for training. By making models more adaptable, it is opening up new possibilities for AI in resource-constrained environments.
Federated Learning
Federated learning is addressing the growing concern of data privacy in machine learning. This technique allows models to be trained across multiple decentralized devices or servers holding local data samples. Key developments include:
- Enhanced algorithms for efficient distributed learning
- Improved techniques for ensuring data privacy and security
- Methods for dealing with non-uniform and biased data distributions
This approach is particularly valuable in scenarios where data cannot be centralized due to privacy concerns or regulations. In healthcare, federated learning has enabled collaborative research on clinical data without compromising patient privacy. Meanwhile, mobile app developers are using it to improve user experiences while ensuring personal data remains on users' devices.
Federated learning is not only preserving privacy but also enabling machine learning in scenarios where data collection was considered challenging or impossible. This is opening new frontiers in personalized AI services and collaborative research.
These advanced research areas drive innovation by tackling key challenges in data generation, adaptive learning, and privacy protection. As they continue to evolve, they promise to expand the capabilities and applications of AI across various domains.
Specialized Topics for 2024
Machine learning is advancing by leaps and bounds, with three key areas playing a crucial role: Quantum Machine Learning, Explainable AI, and AI in Healthcare. These emerging fields are addressing significant challenges and unlocking new AI-based applications.
Quantum Machine Learning
Quantum Machine Learning combines quantum computing with machine learning to enhance AI capabilities. This emerging field leverages the power of quantum computers to improve performance in several areas, including:
- Quantum techniques to accelerate data processing and pattern recognition.
- Hybrid systems that use both classical and quantum computing to tackle complex tasks.
- Innovative ideas from quantum science that enhance classical computing technologies.
Quantum ML has the potential to address complex problems more quickly than traditional computers. This could benefit fields such as financial planning, drug discovery, and data security. While still in its early stages, as quantum computing continue to advance, we can expect to see more practical applications emerge.
Explainable AI
This is about making AI easier to understand. As AI makes more important decisions, people need to know how it thinks. New improvements include:
- Better ways to show how AI makes decisions
- Easy-to-understand AI models
- Methods to explain AI choices in simple words
This is really important in areas like healthcare, banking, and law. It helps people trust AI more because they can see how it works. It also helps find and fix unfair AI decisions.
By improving transparency, AI can be utilized more effectively in critical tasks, fostering improved collaboration between humans and intelligent systems.
AI in Healthcare
AI is increasingly being used in healthcare. It is changing the way how doctors diagnose and treat patients. Key research areas include:
- Better ways to look at medical images and detect diseases.
- Predicting patient outcomes and identifying effective treatments
- Creating custom treatment plans for each patient
AI is assisting doctors in making quicker decisions and providing more accurate diagnoses from scans and lab tests. It is also playing a crucial role in predicting health risks and identifying the most effective treatments.
In drug research, AI is accelerating the discovery of new medicines. Furthermore, AI is transforming patient care by enabling smart devices to work together to continuously monitor health and detect issues early.
These advancements represent some of the most exciting areas of AI research. They are addressing significant challenges in computing, improving the interpretability of AI, and expanding its application in critical fields like healthcare. As these innovations progress, AI's capabilities will continue to grow further.
Emerging Trends and Future Directions
As AI and ML grow, new ideas are coming into the spotlight. Let's look at three big trends in AI and ML research:
Safer AI
People are working on making AI systems that cannot be fooled easily. They're doing this by:
- Finding ways to spot fake data that might mislead AI.
- Building AI that can handle odd or inaccurate information.
- Testing AI extensively to ensure it works smoothly even in challenging situations.
This is important because we need to rely on AI when it is used for important jobs like driving cars or assisting physicians.
AI Stopping Cyber Attacks
AI is currently being used to protect computers and networks from bad actors. This is being achieved by:
- Looking for unusual activities on computer networks
- Trying to guess what new attacks might look like
- Responding swiftly when a problem arises
AI can rapidly process large chunks of data, helping it identify red-flags that people might miss. Through learning, AI can also recognize new types of attacks.
AI Helping the Environment
AI is currently being used to protect our planet's natural environment. Examples include:
- Using AI to save energy in buildings and factories
- Looking at pictures from space to check on forests and animals
- Finding ways for trucks and cars to consume less fuel
AI analyzes complex information and finds useful patterns. This helps people make more eco-friendly choices for our planet.
These emerging research trends indicate that AI and ML are rapidly advancing in areas such as safety improvement, cybercrime prevention, and enhancing environmental outcomes. As these innovations advance, AI will play an increasingly crucial role in shaping a better future for humanity.
Summary
ML research topics in 2024 span a diverse spectrum of domains. Each one has a significant role in propelling innovation to shape a better future. From AI that learns quickly to its applications in healthcare, researchers are at the forefront of managing some of society's most pressing needs and challenges. The primary areas that researchers should concentrate on:
- AI that learns and adapts quickly
- Integrating AI with quantum computing
- Developing explainable AI systems
- Strengthening AI security to safeguard against vulnerabilities
- Advancing AI applications in healthcare
- Using AI to address environmental issues
Platforms such as the Cureus Journal of Computer Science are important for research and collaboration. They enable researchers to share their findings and make new ML research available to everyone without any paywall barriers.
We encourage researchers to focus on impactful projects. Areas such as healthcare and sustainability are particularly valuable. These efforts can lead to significant advancements for society.